Proactive steps to preserve your academic reputation in an era of accountability and data-driven res
- Jason Thatcher
- Aug 15, 2022
- 2 min read
You may have heard of James E. Hunton. A once-famous accounting scholar, evidence suggests that his work was based on fabricated data. To date, 25 or more of Hunton’s papers, involving dozens of collaborators, have been formally retracted due to fraud.
He came to mind recently, when allegations surfaced that Dan Ariely, a famous psychology professor, and behavioral economist, relied on ambiguous and possibly fraudulent data.
In Hunton’s case, his employing university cleared his collaborators. As best I can tell, Hunton has disappeared – no longer in academe and untraceable – implicitly acknowledging his guilt?
In Ariely’s case, he acknowledged data problems, cleared his collaborators, and blamed the sponsoring company. He continues to conduct research.
These cases caused me to ask – how could their collaborators not know?
They didn’t know, because academics are trained to trust.
In my own work, I extend almost blind trust to my collaborators. I often help write papers without confirming the data’s provenance, independently verifying data analysis, and sometimes, not even seeing the data.
While Ariely escaped, seemingly unscathed, I can’t, and most academics can’t afford the damage that accompanies alleged academic fraud.
So what to do?
First, know your coauthors. Take time to learn about potential collaborators, carefully assess their published work, and quietly ask around about their conduct. If you feel comfortable, build a working relationship.
Second, know the data’s source. Even if it requires a non-disclosure agreement, you need to be able to answer questions about the data’s source.
If you were on board as a project started, you likely know how the data’s provenance. If you joined later, ask hard questions about the data, how it was collected, and where. If you adhered here to my first step, this should not be a problem.
Third, verify the data. Ask for a copy of the dataset and familiarize yourself with its content. This will make it easier to work on future analysis and let you rest a little more easily.
Fourth, curate the data. Curate a copy of the original, unedited, untransformed dataset as the project begins. That way, after a paper, is accepted, it’s no problem to share the data.
Fifth, archive files associated with data analysis. I ask my students to save the scripts used to process and analyze the data. I ask them to take screenshots of important settings. This practice allows you to return to the data, recreate analysis, and answer questions, when necessary.
If you practice these five basic steps, you’ll be better able to navigate the evolving trust-but-verify culture of academic life - and sleep at night!
#academiclife#dataprovenance#academicfraud#academicintegrity#data#research#analytics#datacuration#phd#reputationmanagement
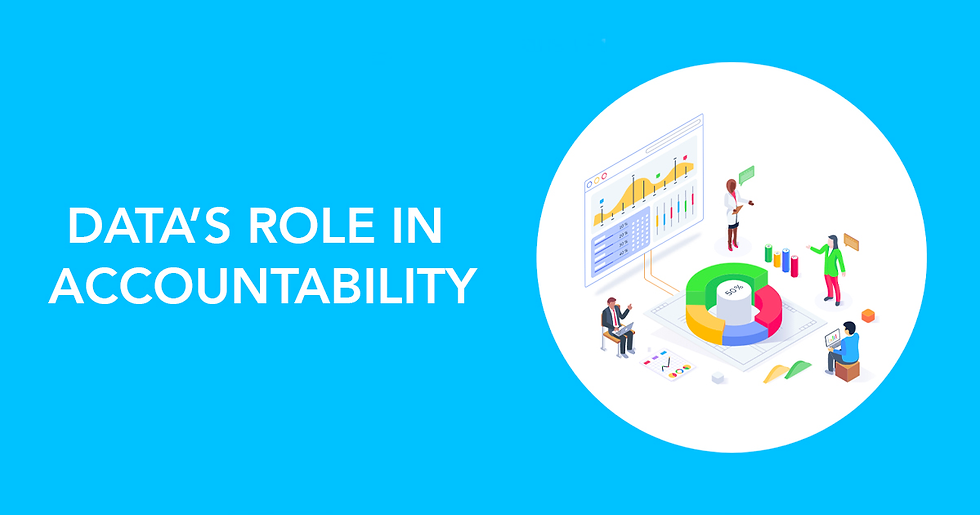
Originally posted on LinkedIn:
Comments